Google orders TSMC to produce the Tensor G5 chip, and upgrades to a 3-nanometer
Development of the Tensor SoC is making continuous progress, breaking Samsung's integrated wafer foundry and packaging testing model.
Since the first Tensor SoC was equipped in the Pixel 6 series in 2021, Google has used Samsung's foundry-produced chips as the core of its mobile phones. However, next year's tenth-generation Pixel will usher in a significant change, with the Tensor G5 expected to become the first Pixel series dedicated chip produced by TSMC.
The Information reported in July last year that Google has reached an agreement with TSMC to produce fully customized Tensor SoCs for Pixel devices. If Google retains the existing naming method, this chip may be called the Tensor G5. Since the foreign media revealed this, the development of the Tensor SoC has been making continuous progress, including the news that the test order was won by Kyun Yuan Electronics, breaking Samsung's integrated wafer foundry and packaging testing model.
Another media outlet, Business Korea, recently reported that Google will use TSMC's 3-nanometer process for the Tensor G5 to be launched next year, which is expected to greatly enhance the performance level of the Pixel series. The currently available Pixel 8 series uses the Tensor G3, which is built with Samsung's 4-nanometer process. By the second half of 2025, switching to a 3-nanometer process is inevitable.
This is not surprising, as Apple started using a 3-nanometer process from the iPhone 15 Pro series last year. More importantly, it is expected that Qualcomm and MediaTek's next-generation chips will follow suit, and the non-Apple camp's Tensor G5 will not have a unique process advantage.
Advertisement
In addition, the report from Business Korea also discussed that Samsung is working hard to solve the issues of yield and power consumption. The upcoming Exynos 2500 chip claims to have power consumption and heat dissipation performance about 10% to 20% lower than TSMC's 3-nanometer process.
Apple has been equipped with its self-developed A-series chips since the iPhone 4 and has expanded its customized M-series chips to the entire Mac series. It has been nearly a year since the development of 3-nanometer process chips for the iPhone and Mac. Android camp competitors have just started to get involved in this technology, and the Tensor SoC's transfer to TSMC production is expected to bring a perceptible upgrade to the new Pixel devices.Google's New Generation Cloud AI Chip
Previously, Google introduced the TPUv5p, which is its latest generation of cloud AI chips and also the most powerful and cost-effective chip to date. Each TPUv5p Pod contains up to 8,960 chips, interconnected through high-bandwidth chip-to-chip connections to achieve fast data transfer and optimal performance.
The new generation TPUv5p excels in AI performance, offering 459 teraFLOPS of bfloat16 performance or 918 teraOPS of Int8 performance, equipped with 95GB of high-bandwidth memory and a data transfer rate of 2.76TB/s. Compared to the previous TPUv4, the TPUv5p has doubled the number of floating-point operations and tripled the high memory bandwidth, which has attracted widespread attention in the field of artificial intelligence.
In addition, the TPUv5p has also improved the training speed of large language models (LLM) by 2.8 times, which is about 50% faster than the previous TPUv5e. Google has also increased the computational power, making the scalability of TPUv5p four times higher than TPUv4. Overall, the TPUv5p has the following improvements compared to TPUv4: the number of floating-point operations has increased by 2 times, memory capacity has increased by 3 times, LLM training speed has improved by 2.8 times, embedding-intensive model training speed has improved by 1.9 times, bandwidth has increased by 2.25 times, and chip-to-chip interconnect bandwidth has doubled.
Google has achieved significant success in the field of AI and attributes it to excellent hardware and software resources. Google's cloud AI supercomputer is a set of elements that work together to achieve modern AI workloads. Google integrates performance-optimized computing, optimal storage, and liquid cooling to fully utilize its huge computing power, thus achieving industry-leading performance.
In terms of software, Google has strengthened support for popular machine learning frameworks (such as JAX, TensorFlow, and PyTorch) and provided some powerful tools and compilers. These tools and compilers can optimize distributed architectures, making the development and training of complex models on different hardware platforms more efficient and easier to use. Google has also developed multi-chip training and multi-host inference software to simplify the management of scaling, training, and serving workloads.
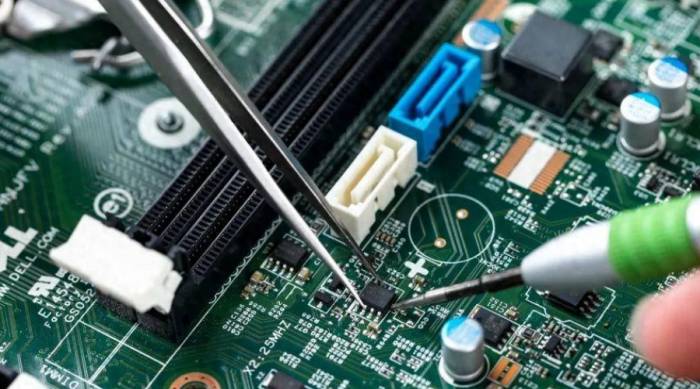
Google's revolutionary approach to artificial intelligence is strongly supported by hardware and software elements that will break various limitations in the industry. The newly released cloud AI chip TPUv5p and Google's AI supercomputer will bring more possibilities and opportunities for ongoing AI development. It is foreseeable that these advanced technologies will further intensify competition and promote the development of the field of artificial intelligence.
Google's new generation cloud AI chip TPUv5p has excellent performance. Compared with the previous TPUv4, TPUv5p has made significant improvements in many aspects.
Firstly, the number of floating-point operations of TPUv5p has doubled. It can provide 459 teraFLOPS of bfloat16 performance or 918 teraOPS of Int8 performance, greatly increasing the speed of computation. This is very helpful for handling complex computing tasks and large-scale machine learning models.
Secondly, the memory capacity of TPUv5p is three times higher than that of TPUv4. It is equipped with 95GB of high-bandwidth memory, which can access and store data faster. This is crucial for handling large-scale datasets and complex model training.Thirdly, the TPUv5p has demonstrated a 2.8-fold generational improvement in the training speed of large language models (LLMs). This is very helpful for tasks such as natural language processing and machine translation, as it can accelerate the training speed of models and enhance work efficiency.
In addition, the TPUv5p has also made significant progress in the training speed of embedding-dense models, achieving a 1.9-fold increase. This is very beneficial for handling deep learning models and neural network models, as it can improve the training efficiency and accuracy of the models.
Finally, the TPUv5p has also seen a significant improvement in bandwidth and inter-chip interconnect speed. Its bandwidth has increased by 2.25 times, reaching a transfer speed of 2765GB per second. The inter-chip interconnect bandwidth is twice the original, reaching a transfer speed of 4800Gbps per chip. This can improve the efficiency and speed of data transmission, thereby enhancing the overall performance.