The price war of large models has started, can multi-core hybrid become a breakt
Recently, several major model companies in China have successively lowered the prices of their related products.
Starting in early May, out of 9 domestic major model companies that released new content, 7 announced price reductions. These include: Depth Quest, ZhiPu AI, ByteDance, Alibaba Cloud, Baidu, iFLYTEK, and Tencent Cloud, involving a total of 21 models. Some large factories have even adopted the slogan of "free mode."
01
The price war of large models is becoming more and more intense
On May 6th, Depth Quest, founded by the well-known private equity giant Huanfang Quantitative, released the second generation of MoE model DeepSeek-V2. MoE model, that is, the mixed expert model, breaks down complex tasks into sub-tasks and hands them over to the appropriate "expert" models for processing, improving accuracy and reasoning efficiency. At the same time of model iteration, Depth Quest reduced the API call pricing to 1 yuan for every 1 million tokens input and 2 yuan for output, which is only one percent of GPT-4-Turbo.
On May 11th, ZhiPu AI followed up and announced a 80% reduction in the call price of its GLM-3 Turbo model. It was reduced from 5 yuan/1 million tokens to 1 yuan/1 million tokens. 1 yuan can purchase 1 million tokens.
On May 15th, ByteDance's Dou Bao large model was officially opened to the outside world, releasing a very low price compared to the industry, and the price unit directly changed from "yuan" to "fen". The Dou Bao large model family includes two general models: Dou Bao General Model PRO version and lite version. Among them, the Dou Bao General Model pro-32k version, the inference input price is 0.0008 yuan/1,000 tokens, which is 99.3% lower than the industry price. The lite version is 0.0003 yuan/1,000 tokens.
Advertisement
Subsequently, on May 21st, Alibaba Cloud also issued a price reduction notice, and the 9 main large models of Tongyi were significantly reduced in price. Among them, the API input price of the main model of Tongyi Qwen-Long at the level of GPT-4, which is the main model of Tongyi Qwen-Long, was directly reduced by 97% to 0.0005 yuan/1,000 tokens. 1 yuan can buy 2 million tokens, which is equivalent to the amount of text in five "Xinhua Dictionary".
On the same day, Baidu even announced that the two main models of the Wenxin large model, ENIRE Speed and ENIRE Lite, are completely free. iFLYTEK also announced on the 22nd that the API of Xunfei Xinghuo Lite version is permanently free, and the price of Xunfei Xinghuo Pro/Max API was reduced to 0.21 yuan/10,000 tokens. In addition, Tencent also announced a brand-new large model upgrade plan on the 22nd. One of the main models of Tencent, Hunyuan-lite model, plans to upgrade the total length of API input and output from the current 4k to 256k, and the price is adjusted from 0.008 yuan/1,000 tokens to completely free.In the fierce competition of the AI field, large models are gradually being drawn into the whirlpool of price wars. So, what are the deep-seated reasons driving this change, and how will it profoundly affect the entire industry ecosystem?
02
What is the essence of price reduction?
Price wars are beneficial for giants to seize the market
The current industry price war can be seen as a derivative result of the "hundred model battle." When the large model craze was at its peak, a large model seemed to "pop out" almost every other day. As of November 30, 2023, there are at least 200 large model manufacturers in China that have launched their respective large models.
The current competition among large models has long surpassed the realm of technology, and it is more of a competition at the ecosystem level, specifically reflected in the number of applications, the number of plugins, the number of developers, and users, etc.
It should be noted that the current large model market is quite limited in space, and most large model apps have already begun to face the dilemma of sluggish user growth, including the highly regarded OpenAI. Therefore, price reduction is one of the ways for these large manufacturers to gain more market share.
In addition, from a price perspective, the prices of some startups are inherently low. Therefore, in response to the current tech giants' price reductions, those AI startups have mostly chosen not to follow suit. Some investors involved in AI large model investments have said, "This round of price reduction has a greater impact on some startups' TO B models." Because in the past, many companies chose to cooperate with startups mainly because the startups' APIs were cheaper than those of large manufacturers, but now there is basically no possibility of being cheaper than large manufacturers, which means that the B-end commercialization model of startups no longer exists.
For these startups, if they cannot find a new way out, they may face a life-or-death test.The capability gap of entry-level, lightweight large text models is not significant.
Semiconductor industry observations have found that in this wave of price reductions, the models that have been reduced in price are mainly entry-level, lightweight large text models, while high-performance and multimodal models in verticals such as image recognition and speech recognition have not adjusted their prices.
The technology and capabilities of these entry-level, lightweight large text models have converged in various aspects, and the technical barriers between manufacturers are not significant, so price competition has become the main means of competition between them.
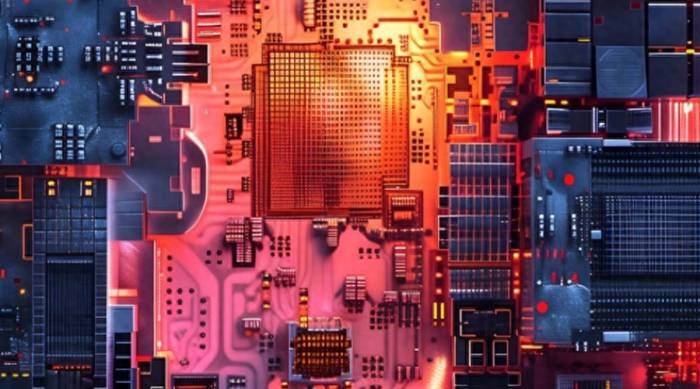
According to the large model open source open evaluation system "Sinan (OpenCompass2.0)" released by the Shanghai Artificial Intelligence Laboratory, complex reasoning-related capabilities are a common challenge for large models. Domestic large models still have a gap compared to GPT-4, which is a key capability required for the landing of large models in reliable scenarios such as finance and industry. However, in the Chinese scene, the latest domestic large models have shown unique advantages, especially in language and knowledge dimensions, approaching the level of GPT-4 Turbo.
The marginal benefits of large models are continuing to decline.
Dr. Gary Marcus mentioned in the article "Evidence that LLMs are reaching a point of diminishing returns — and what that might mean" that from GPT-2 to GPT-4 and even GPT-4 Turbo, there have been signs of diminishing performance.
Dr. Gary Marcus said: "Since the release of GPT-4, many models have converged significantly at the GPT-4 performance level, but there is no obviously leading model."
Under the background of diminishing returns, it means that the actual cost for developers to handle the same tasks is rising. In the market environment where the prospects of AI innovation commercialization are still unclear, in order to retain existing users, large model manufacturers must provide attractive countermeasures. This includes providing smaller models, such as Google's Gemini 1.5 Flash. Another means is direct price reduction.High investment, multi-core hybridization may be helpful
The core of artificial intelligence is computing power, which is mainly divided into two parts: training computing power and inference computing power.
At present, the demand for training computing power is very high. According to data from last year, the public data of ChatGPT shows that its entire training computing power consumption is very large, reaching 3640PF-days. Converted to Nvidia A100 chips, its single card computing power is equivalent to 0.6P of computing power. Under ideal conditions, a total of about 6000 are needed. Considering the loss of interconnection, 10,000 A100 chips are needed as the foundation of computing power. At the price of 100,000 RMB per A100 chip, the hardware investment scale of computing power reaches 1 billion RMB. The inference computing power is mainly Nvidia T4 cards, and the inference cost is about one-third of the training cost.
In addition to the cost of computing power, there are also a series of costs that come with it, such as storage, inference, operation and maintenance, and application.
So how to solve the most concerned "cost reduction and efficiency improvement" problem of most enterprises at present? In addition to optimizing the model, innovative ideas at the hardware level cannot be ignored. Recently, many experts and technical personnel in the industry have started to focus on the concept of multi-core hybridization, trying to bring higher performance and lower costs to enterprises through this strategy.
So what exactly is multi-core hybridization? How does it provide a better solution in the current shortage of AI model computing power?
Multi-core hybridization mainly involves combining different types, different functions, or different process architecture chips in hardware design or application to form a hybrid computing system or solution. The aforementioned current basic large model training requires the largest AI computing power cluster scale, which has gradually increased from a single cluster of thousands of cards to a ten thousand card level. At the same time, many intelligent computing centers have deployed GPU clusters, which are usually several to hundreds of servers, and it is difficult to meet the needs of future industry large model training.
Therefore, on the basis of the existing AI computing power cluster, building a single cluster composed of different chips such as Kunlun Core and Ascend to provide greater AI computing power for large model training has become a natural choice.
What are the advantages of multi-core hybridization?
First, by distributing computing tasks to multiple GPUs, the training speed of the model can be significantly accelerated. Multi-GPU parallel training can also reduce the time waste caused by computational bottlenecks in single-GPU training, thereby improving training efficiency.Second, training with multiple GPUs can process more data simultaneously, thereby improving memory utilization.
Third, the construction of such a hybrid cluster can effectively reduce costs. After all, compared to Nvidia's A100/H100 series GPUs, GPUs from other brands are more affordable.
However, if this plan were as easy to implement as we imagine, it would have already been adopted by many industry giants. Let's take a closer look at the difficulties in implementing this plan.
04
What issues need to be addressed for multi-core hybridization?
To build a cluster capable of efficiently training large models, it is necessary to establish efficient interconnectivity between cards and machines, divide the large model training tasks according to appropriate parallel strategies into GPU cards, and finally, through various optimization methods, accelerate the computational efficiency of GPUs for operators to complete the training of large models.
However, it is difficult to interconnect different chips because the physical connection methods, parallel strategies, and AI acceleration suites of Nvidia GPUs, Kunlun chips, and Ascend 910B are all different.
Firstly, in terms of interconnectivity, the 8 GPU cards within a single server are connected via NVLink. GPU cards between different servers are connected via RDMA networks.
In the past, we have seen many introductions to Nvidia GPUs and the CUDA moat. Indeed, after years of investment, they have established an insurmountable advantage. But in addition to this, as mentioned above, Nvidia has many invisible moats, and NVLink is one of them, a technology that provides high-speed connections for GPU-to-GPU interconnectivity.
In the current era where Moore's Law is gradually becoming obsolete, but the demand for computing power is increasing, such interconnectivity is particularly necessary.NVIDIA's official website states that NVLink is the world's first high-speed GPU interconnect technology, providing an alternative choice for multi-GPU systems, with significant speed improvements compared to traditional PCI-E solutions. By connecting two NVIDIA GPUs with NVLink, memory and performance can be flexibly adjusted to meet the demands of the highest workloads in professional visual computing.
The Kunlun Core server is internally connected through XPU Link, and servers are connected to each other via standard RDMA network cards, with cards communicating with each other using the XCCL communication library. The Ascend 910B server is internally connected through HCCS, and servers are connected to each other via Huawei's self-developed built-in RDMA, with cards communicating with each other using the HCCL communication library.
Secondly, in terms of parallel strategies, NVIDIA GPUs and Kunlun Cores adopt a deployment method of 8 cards per machine, while Ascend 910B is divided into 2 communication groups of 8 cards within the machine. This means that different cluster topologies are formed under the AI framework, and targeted distributed parallel strategies need to be formulated.
Finally, in terms of AI acceleration suites, due to differences in computing power, memory size, I/O throughput, communication libraries, etc., of chips such as Kunlun Core, Ascend, etc., specific optimizations need to be made for specific chips. The final result is that each chip has its own corresponding operator library and corresponding acceleration strategy.
05
Which manufacturers have started to try the water?
It is worth noting that recently, an alliance composed of leading technology companies such as AMD, Broadcom, Cisco, Google, Hewlett Packard Enterprise (HPE), Intel, Meta, and Microsoft has announced the establishment of the Super Accelerator Link (UALink) Promotion Association. The plan aims to develop an open industry standard to promote high-speed, low-latency communication of AI systems in data centers.
Faced with the growing AI workloads, these tech giants are all urgently in need of ultra-high-performance interconnects.
Baidu is also building a multi-core hybrid training AI cluster. Baidu Bai Ge's multi-core hybrid training solution shields the underlying complex heterogeneous environment, integrating various chips into a large cluster, which can unify the existing different computing power, integrate and exert the maximum efficiency of these computing powers, and support larger model training tasks. At the same time, it supports the rapid integration of new resources to meet the needs of future business growth. The solution is not only provided through Baidu's public cloud but also delivered through the ABC Stack private cloud.
Previously, Shen Dou, Executive Vice President of Baidu Group and President of Baidu's Intelligent Cloud Business Group, said that in terms of "one cloud with multiple cores," Baidu Bai Ge is compatible with mainstream AI chips at home and abroad such as Kunlun Core, Ascend, Hygon DCU, NVIDIA, and Intel, supporting the mixed use of chips from different manufacturers in the same intelligent computing cluster, maximizing the shielding of differences between hardware, helping enterprises get rid of dependence on a single chip, and creating a more cost-effective, safer, and more flexible supply chain system. In multi-core hybrid training tasks, Bai Ge can maximize the utilization rate of a single chip, chip-to-chip communication efficiency, and the overall performance of the cluster, with performance loss of no more than 3% at the scale of a hundred cards and no more than 5% at the scale of a thousand cards, both of which are at the highest level in the country.Recently, the open-source large model parallel training framework, FlagScale, has been comprehensively upgraded. The Zhiyuan team, in collaboration with the TianShu Zhixin team, has achieved heterogeneous hybrid training of a single large model task on a cluster with "NVIDIA chips + other AI chips", and has verified the effectiveness of heterogeneous hybrid training on different architecture chips on a 70B large model. At the same time, in order to accelerate the use of various AI chips in large model training scenarios, Zhiyuan actively explores efficient and flexible chip adaptation solutions. Through in-depth cooperation with hardware manufacturers, FlagScale has adapted the large-scale training of the Aquila2 series large models on multiple AI chips from 6 different manufacturers.
Due to the different interconnect protocols between cards from different manufacturers, in order to achieve high-speed interconnection of "NVIDIA chips + other AI chips", the Zhiyuan team collaborated with TianShu Zhixin to optimize TianShu Zhixin's iXCCL communication library, making it compatible with NVIDIA NCCL in terms of communication primitive operations and API interfaces. Then, the framework was compiled and linked to the same iXCCL communication library, thereby achieving efficient communication between heterogeneous computing power chips without the awareness of users and AI frameworks, and thus achieving heterogeneous training of different architecture chips. At the same time, the two parties also collaborated to optimize the allocation method of pipeline parallelism, and configured different pipeline parallelism strategies for different chips according to the differences in chip computing power, memory bandwidth, and memory capacity, so as to fully utilize the performance of different chips during the training process, and ultimately took the lead in implementing an efficient training solution for heterogeneous large models with general-purpose GPUs.
Opportunities for domestic GPU manufacturers
Multi-core hybrid technology allows different architectures and functions of chips to be integrated into a system, providing opportunities for technological innovation for domestic manufacturers. By integrating and optimizing the performance of different chips, more efficient and flexible solutions can be developed.
Multi-core hybrid technology has brought comprehensive development opportunities for domestic manufacturers. This technology not only promotes technological innovation and meets the growing market demand for high-performance, low-power chips, but also promotes collaborative cooperation between upstream and downstream of the industry chain, and strengthens the overall competitiveness of the industry. At the same time, national policy support also provides a strong guarantee for the development of domestic manufacturers in the field of multi-core hybrid technology. Domestic manufacturers should seize this opportunity, increase R&D efforts, promote breakthroughs and applications of multi-core hybrid technology, and improve the technical level and market competitiveness of domestic chips.